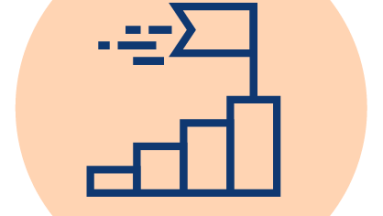
9 Ways to Improve Your Next Data Visualization
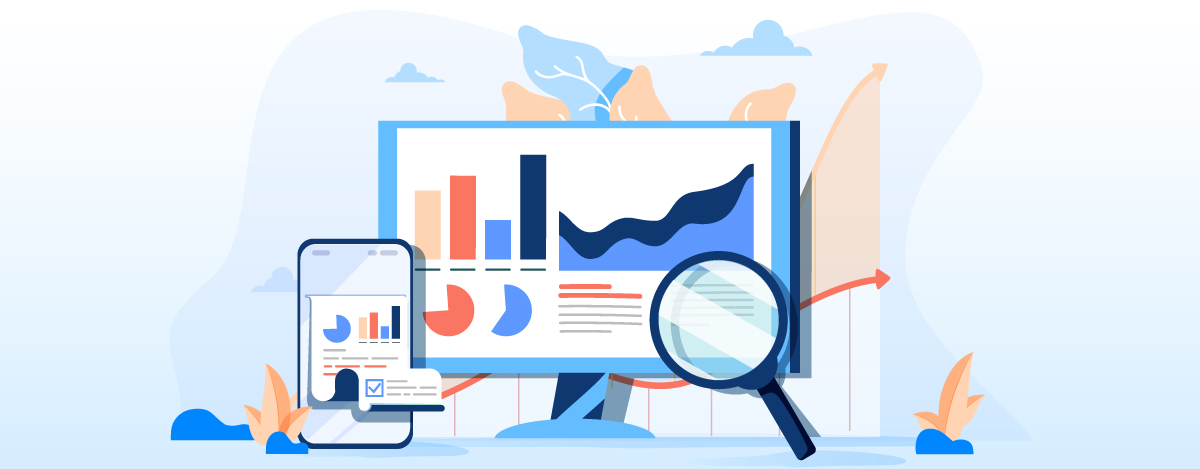
Transforming a dataset from numbers on a spreadsheet to an eye-popping visualization is not always a one-and-done process. It’s an undertaking that requires trial and error and can sometimes surprise designers with new insights about the data during the course of design.
While creating a data visualization should not be a difficult task, there are strategies designers can employ to ensure the end result sends a clear and compelling message — the most important component of a data visualization. Designers can include elements such as color, text, animation and other features within their charts to ensure their points are effectively conveyed.
Lisa Rost, designer for Berlin-based data visualization platform Datawrapper, noted that data is a subjective medium.
“There’s always this question of, ‘Do we really want people to use these tactics, or is data something that we want to be like just rational, just objective, unbiased?’” Rost said. “And no, it’s actually not. In the best case, people understand data as a medium like text. How you communicate it has a huge impact on how you understand it, on the reader.”
The goal of a visualization will determine its appearance: simple or complex, colorful or plain, animated or static. The following guidelines can help your next data visualization resonate with your audience, no matter the size.
Nine Considerations for Your Next Data Visualization
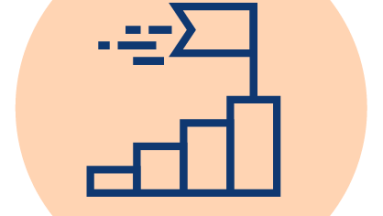
Decide where your visualization falls on the spectrum between exploration (charts used for analytics) and communication (charts used in presentations or publications). Then determine what message you want the audience to take away from the visualization.
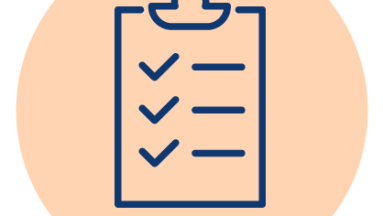
2. Clean up and understand your dataset.
Make sure you thoroughly understand the data you’re trying to explain and you have a clean set of data to work with. Some early analysis may be required before your data can be visualized, and if you’re working with a large dataset, it could be helpful to home in on particular points.
“It’s more like a cycle because you don’t know the goal until you understand the data, so you do need to do some analysis of the data first,” Rost said.
Exploratory data analysis, or EDA, is a good approach to take when working with a dataset for first time. The approaches you take here and the trends you discover may influence the way you ultimately visualize your data.
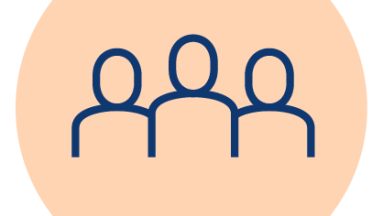
3. Know your audience.
Your audience will help determine what elements to use within the visualization, such as text, colors and other effects. Charts used for internal stakeholders will look different from those designed for external audiences. Consider how familiar the audience is with the subject and whether they might need extra explanation as to why what they’re seeing is important.
“What are they bringing to the table?” said Nicolas Kruchten, vice president of product for Plotly, a developer of data analytics and visualization tools in Montreal. “Do they know how to read a sophisticated chart? Do they have an opinion on the subject? Do they know anything about the subject?”
Such questions will help shape the chart and its extra design elements.
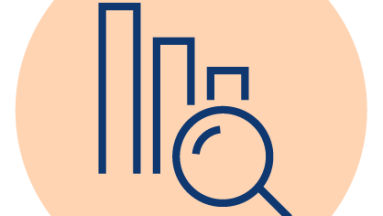
4. Choose a type of chart.
Experimentation is part of the process in selecting a chart type, so try visualizing your data in different ways.
“It’s … something that can change all over again,” Rost said. “It’s not like a clear linear process.”
There are dozens of types of charts: line graphs, bar charts, scatterplots, maps — the list goes on. Testing out different types of visualizations can allow you to see what best conveys your point.
Understanding the reasoning behind using certain chart types can help you choose the best visualization for your audience. As Andrew Abela, founding dean of Catholic University of America’s School of Business & Economics, explained in a flow chart on chart suggestions, data visualizations can generally be grouped into one of four usages: comparison, relationship, distribution or composition. Some charts are more effective than others, depending on the type of information they aim to communicate. Bar charts, line charts and circular area charts work well for comparing variables; scatter plots are most effective in displaying relationships between variables; histograms and scatter plots can best visualize distribution; and stacked bar charts, stacked area charts and pie charts are among the best for displaying the composition of a dataset.
“The more experience you have in a sphere, and the more charts, et cetera, you have created, the more you intuitively know which chart types would work well,” Rost said. “There are lots of chart types that will make sense, that will show the data, but they won’t show what you actually want to show.”
Making a single chart doesn’t need to be a laborious task. If you’re efficient, it won’t matter if you get it “wrong” the first time — or the first hundred times.
“When I make a chart, I’m expecting to make a couple hundred iterations — literally,” Kruchten said.
Going through the process of creating and recreating visualizations can also answer key questions about the data and your main message. You may decide to highlight different points that you hadn’t noticed before visualizing the data in a variety of forms.
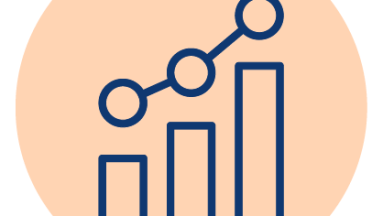
5. Don’t try to pack too much into one chart.
Don’t limit yourself to one visualization. If two or more charts illustrate your point better than one — and the information is easier to digest — use them both.
“There’s nothing wrong with showing the same data twice in two different ways,” Kruchten said.
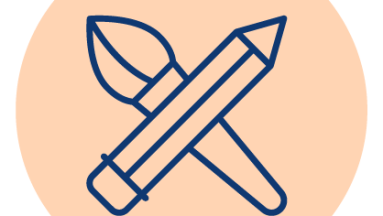
6. Map the data to visual variables.
“Your most important data should be encoded in either the length of the bar or the position of the point on your chart rather than the color,” Kruchten said. “Being aware of the hierarchy of the visual variables and how the brain processes them is an important part of sort of choosing a chart.”
William S. Cleveland and Robert McGill’s 1985 paper “Graphical Perception and Graphical Methods for Analyzing Scientific Data” established the importance of different types of visual elements based on how accurately the human brain perceives visual markers. Designers still refer to this hierarchy to increase the accuracy with which their data visualizations are perceived:
- Position along a common scale
- Positions along identical but nonaligned scales
- Length
- Angle, slope
- Area
- Volume, density, color saturation
- Color hue
As you’re adding color and determining size and scale, use common sense and defer to cultural norms. The palette should reinforce the audience’s expectations associated with particular colors.
“Red generally means ‘bad’ or ‘stop’ in Western cultures; green means ‘good’ and ‘go.’ But not the same thing in other cultures,” Kruchten said.
Big visual elements should indicate more importance or bigger quantities.
In more elaborate visualizations, consider using animation to convey emotion and build tension. Animation allows readers to see how trends unfold over time, and can be a compelling storytelling tool for darker subjects, such as rising death tolls.
“Animation is very powerful there and, I would say, more powerful than color,” Rost said.
If your visualization is for a public audience, fit the fonts, colors and other design elements to the publication or presentation where it will appear. Refer to style guides if the graphic is being used for publication.
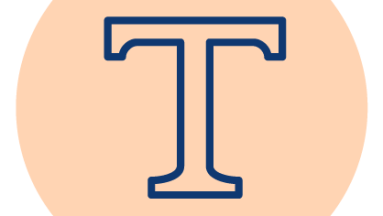
7. Text is “totally underrated.” Use It.
After working with a dataset for days, weeks or months, it can be easy for designers and data scientists to skimp on explanations if they forget their audience has never seen the data.
“Text is a totally underrated design element,” Rost said. “The huge challenge is to see the data visualization with the eyes of someone who has never seen it before and describe in detail what people can actually see and why it’s important.”
Most visualizations could benefit from text, especially when the audience isn’t familiar with the dataset or topic.
“You shouldn’t be afraid of having long textual explanations beside [or] inside your chart. To the extent that your chart is used for communication, you should have as much text as is required to get the point across by really thinking critically through the eyes of your audience,” Kruchten said. “If you’re not talking to an expert audience, then you need to help them along.”
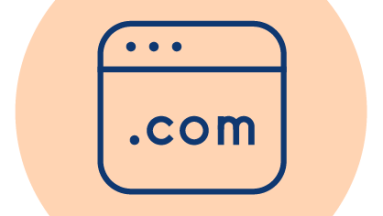
8. Include the source of the data and link to the original dataset, if possible.
Be critical of and honest about your datasource from the start. Know where the data came from, how it was collected, what was omitted and what conclusions you can reasonably draw from it.
“Help your audience draw the correct conclusions,” Kuchten said. “You have a certain responsibility to pass along all of the caveats of the data, essentially.”
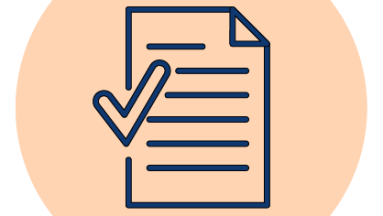
9. Know the rules — so you know when to break them.
“There are a lot of dos and don’ts out there, like, ‘don’t use pie charts,’ ‘don’t use 3D.’ Some of those are a reasonable starting point, but … people make 3D charts sometimes for good reason; people make pie charts because people expect pie charts,” Kruchten said. “Instead of knowing the rules and not doing it, try and ask yourself why it’s not recommended and if there’s some way of getting around that.”