Five Women in Data Science Share Advice on Taking Risks, Building Inclusivity and Harnessing ‘Street Cred’ in Tech
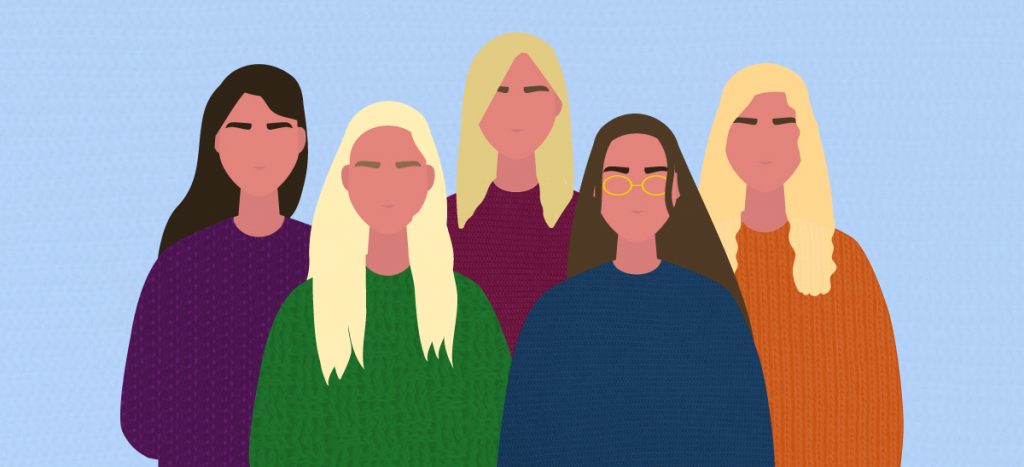
The demand for science, technology, engineering and math occupations is expected to grow during the next decade. But even with the growth in opportunities, women remain underrepresented in these fields. The U.S. Bureau of Labor Statistics estimates that only 16.5% of the engineering and architecture workforce and 25.2% of employees in computer and mathematical jobs were held by women in 2020.
We spoke with five female data scientists about the current landscape of opportunities for women in big data. Read on to learn about these women—Jana Eggers, Claudia Perlich, Lillian Pierson, Jennifer Priestley and Sarah Aerni—as well as their experiences working in the field and what it takes to be successful.
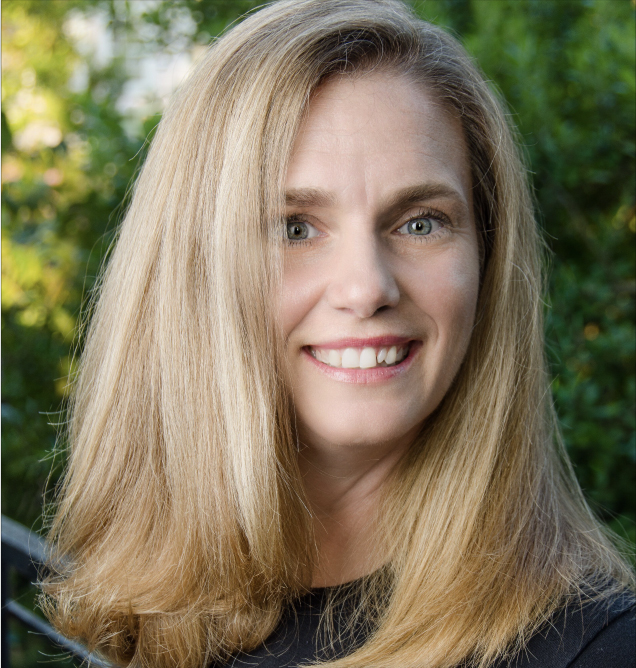
Jana Eggers, Nara Logics
Jana Eggers is CEO of the artificial intelligence company Nara Logics. An AI practitioner, Jana focuses on practical applications of AI for business and problem-solving. Her previous experience includes work at Lycos, American Airlines, Los Alamos National Laboratory, Intuit and a number of startups. A speaker and mentor, Jana also sits on the digital advisory board for Fannie Mae. She holds a bachelor’s degree in math and computer studies from Hendrix College.
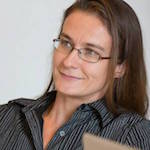
Claudia Perlich, Dstillery
Claudia Perlich, PhD, serves as an advisor at Dstillery where she designs, develops, analyzes and optimizes the machine learning that drives digital advertising. An active industry speaker and frequent contributor to academic and industry publications, Claudia won the Advertising Research Foundation’s Grand Innovation Award, was named Innovator of the Year in the annual Stevie Awards, was selected as a member of the Crain’s New York Business annual 40 Under 40 list, made WIRED’s Smart List, and was listed among Fast Company’s 100 Most Creative People. She has published more than 50 scientific articles and holds multiple patents in machine learning. Claudia has a doctorate in Information Systems from NYU and worked in the Predictive Modeling Group at IBM’s Watson Research Center, concentrating on data analytics and machine learning for real-world applications. She also teaches in the NYU Stern MBA program.
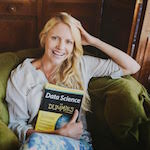
Lillian Pierson, Data-Mania
Lillian Pierson, PE, is a three-time author on the topics of data science, AI and big data. She is CEO of Data-Mania, LLC, a data-strategy consulting boutique that has supported a variety of corporate clients, including Amazon, Intel and the United Nations. She was elected as a Microsoft Regional Director. Through her partnerships with LinkedIn and Wiley, Lillian has trained about one million professionals to develop data skills that generate business value.
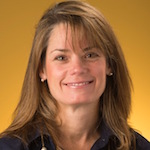
Jennifer Lewis Priestley, Kennesaw State University
Jennifer Lewis Priestley, PhD, is associate dean of the Graduate College at Kennesaw State University and executive director of the university’s Analytics and Data Science Institute. Datanami recognized Jennifer as one of the top 12 “Data Scientists to Watch in 2016.” In 2015, she oversaw the launch of the country’s first doctorate program in data science. Jennifer served as the 2012 and 2015 co-chair of the National Analytics Conference, and the SAS Institute recognized her as the 2012 Distinguished Statistics Professor of the Year. She is a member of the advisory boards for the Southern Data Science Conference and Flock Specialty Finance. Prior to receiving a doctorate in decision sciences from Georgia State, Jennifer worked in the financial services industry. She holds a Master of Business Administration from Penn State and a Bachelor of Science from Georgia Tech.
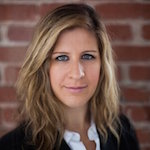
Sarah Aerni, Salesforce
Sarah Aerni, PhD, is director of data science and analytics at Salesforce. She previously led the San Francisco practice at Pivotal as a principal data scientist and data science manager. Sarah also serves as an Apache Software Foundation committee member. She earned her doctorate from Stanford University in biomedical informatics, performing research at the interface of biomedicine and machine learning. She also co-founded a company offering expert services in informatics to both academia and industry.
Which women in data science (or tech in general) inspire you?
“There is nothing better than seeing Lauren in action connecting worlds and creating a tapestry of stories using the backdrop of data.”
Jana: We’re often touted as having a pipeline problem. But I look around, and I think there are amazing women everywhere. Dr. Fei-Fei [Li] at Stanford has been an incredible inspiration. I really love all of the work that’s coming out about biases, something Joy Buolamwini is also working on. Kate Darling is doing inspirational things regarding robot ethics at the MIT Media Lab. And of course, you have people like Joanna Bryson, who’s been working in tech for a long time.
I’m also really excited about the people bringing neuroscience into tech, like Eve Marder, who’s one of the early neuroscience professors; Rebecca Saxe, who does work in early childhood brain development; and Emily Hueske, who conducts research into the rules by which brain circuits make decisions and is one of our advisors at Nara Logics. And I’m inspired by a lot of the new people that are coming in, like Cassie DeWitt, who started doing work in the Detroit public service sector and is now doing some amazing work in data science for the city of New York.
Claudia: Brenda Dietrich was one of my earlier mentors. To me, Brenda embodies the success of a deeply skilled technical expert in a corporate research environment where she took on a leadership position not so much because she desired to manage but because the alternatives would have been much worse for her and all the people who ended up working for her. Brenda is extremely well regarded by both the people working under her and IBM leadership, and she has high external visibility. During her very successful career Brenda has also been one of my role models—and she has raised four children.
I met Kate Crawford two years ago during a two-week stay at the Rockefeller Foundation Bellagio Center. Kate is inspiring in her brilliance, eloquence and passion for addressing some of the less sexy sides of data science, such as privacy concerns or threats to equality caused by algorithms invading our everyday life. Her career is astonishing in terms of academic and political influence. She once had full-time roles at MIT and Microsoft Research while also raising a beautiful baby boy amid all of her professional travel. Kate is another example of a woman with an unbridled “can-do” attitude.
Lauren Moores is a good friend and colleague at Dstillery who entered the world of data after completing a PhD in economics and having taken some time to raise a family. She calls herself a “digital data geek and strategist” who loves “creating stories from dirty and disparate signals and mentoring others to ‘speak’ and think data.” At this point, we share a passion for speaking externally on the facets of emerging data science and for being involved in the creation of the next generation of “geeks” teaching courses at NYU.
Lillian: I am deeply inspired by Sara-Jayne Terp, Heather Leson and Justine Mackinnon. They’ve devoted their lives and careers to using data science and/or technology to help people who are in crisis situations in underprivileged nations.
Sarah: My manager, Hulya Emir Farinas, is easily one of the most talented and inspiring people, male or female, in data science. Hulya is incredibly brilliant, both technically and with her business acumen. She has inspired and greatly educated me. Beyond that, what I find wonderful is that almost any list of top data scientists will contain several women. I feel this cannot be said for many technical fields, so it does not feel like a boys’ club.
How are the opportunities for women in data science? What do you think the future holds?
“I want to come to work and do what I love and be recognized for what I bring to the table and not waste even one thought on the fact that I am a woman. Most successful women I know in the field seem to have this attitude and are very comfortable with themselves and their roles.”
Jana: I feel like we’re just getting started because I think most companies are just realizing they have enough data to make a difference. Before, I think they felt their data was limited and they couldn’t use some of the more advanced tools, but now they know this isn’t just about the Googles and the Facebooks and the Amazons of the world, who clearly have large amounts of data. It’s really about the “mere mortal” companies that can use their own data to improve their business and come up with new offerings.
Here’s an example: I’m sitting here in my office, and I can see a fire extinguisher. There are companies that only service fire extinguishers. Sensors are now being put on those, and companies can actually use that data and sell it to insurance companies, right? That’s a whole new business model, and now they need a data scientist to watch, monitor and understand that data.
There are going to be so many more jobs that allow us to do more with data, which means we’re going to need more data scientists and even data wranglers and data whisperers. It’s not just about the science behind it; it’s about how you handle data and encourage other people to think about it.
Claudia: I see what I would consider a healthy participation of women in the field. They often bring a different intuition to the table and are rising easily into leadership positions. At Dstillery, half of our core data science team are women PhDs from very diverse backgrounds. Similarly, I consistently see nearly half of the students taking my MBA course on data mining for business intelligence at NYU are women. Ultimately, data science is another technical field where women remain statistically a minority, but I do not believe that we need to force the issue and “fight” for a higher female quota. I want to come to work and do what I love and be recognized for what I bring to the table and not waste even one thought on the fact that I am a woman. Most successful women I know in the field seem to have this attitude and are very comfortable with themselves and their roles.
Lillian: The fields of data science and analytics are absolutely exploding with opportunity. For men and women alike, there are plenty of opportunities. The issue for women in this field is getting them trained in STEM (science, technology, engineering and mathematics) areas. We need more women in STEM, period.
Jennifer: I think the current landscape is rich with opportunity, regardless of gender. The trend that excites me the most is that this is really the first career path that is truly interdisciplinary. Courses in applied statistics and applied computer science are filling up with students coming from disciplines where we have not previously seen many students, such as psychology, marketing, finance, economics and sociology. This makes for a particularly rich learning environment where students have to solve problems and work in groups with people who think very differently from them. When an engineering student, a chemistry student and a marketing student all have to solve the same analytics problem, they are learning important latent skills related to teaming, communication and project management.
Sarah: I would say that, luckily, the opportunities for women in data science are the same as those for men. This field benefits from diversity in thinking. There is rarely only one way to approach a problem, and new ideas and input can only lead to more variety in approaches and potentially better outcomes. Not just that, but the consumers of data science exist in every industry and therefore require us to interact with a wide variety of businesses and individuals. Beyond that, it is a field that values a variety of skills, deep technical abilities, hacking, storytelling, visualization and constant learning. This is something I value, and I think it encourages diverse backgrounds as well.
What can be done to encourage more women to pursue data science?
“In fact, many senior data scientists in my network recently agreed that coding tests are not necessarily a requirement for data science interviews.”
Jana: While I don’t think it’s a pipeline problem, I don’t mean that there’s not an industry problem. I think it’s more of an issue with people staying in the industry. There may be a lot of women who are interested, they come in, and data science is still too, in my opinion, akin to the tech industry in that we have some sexism and bias problems. People are not used to someone thinking differently and challenging the way they’re thinking. That’s an issue to consider if you want it to be a more inclusive place. It’s important to recognize people coming from different backgrounds.
I was just working with a woman who was rejoining the workforce after being out of it for six years as she raised kids and did some nonprofit work. She was really struggling because even though she had 12 years of amazing experience in technology and some in data science—and she’s very interested in AI—people weren’t recognizing that. Hiring managers should consider people non-traditionally and understand how different people apply for different jobs. Women are known to not apply for a job that they’re not overqualified for—how many people are you weeding out because of the way you wrote your job description?
Claudia: Many of the women I meet just lack a bit of confidence and need a little nudge to believe in their abilities. Meeting them in low-key social events, such as meetups or specific career advice sessions, has been very rewarding. Coming from other fields, many junior women doubt whether their coding and stats skills are at par to “qualify” for a data science position, but data science roles come in many flavors and not all require master’s level CS knowledge. In fact, many senior data scientists in my network recently agreed that coding tests are not necessarily a requirement for data science interviews.
At Dstillery, we have women PhDs in data-related positions coming from very diverse fields: information systems, physics, neuroscience, economics and genetics. I would tell junior women to get their feet wet and start working on projects either related to their work or school or just on their own. On the other side, I would like to encourage companies to be more risk-taking in their hiring decisions and embrace diverse backgrounds. Additionally, many of the established women happily take on mentorships or speak at events, such as Grace Hopper reaching out and encouraging the next generation.
Sarah: I think many of the existing programs and meetups out there that focus on bringing women together can help inspire more women to pursue data science. I think the best source of encouragement is from women coming together to support each other. Having positive role models helps, but certainly knowing that we can elevate each other is also important.
What types of challenges do women (but not men) in data science face?
“…there is a perception that if you are a female with a manicure and wear jewelry, then you must not know how to program or understand advanced analytics. But a well-groomed guy carrying a cappuccino cup gets immediate respect. I don’t get it. I think that stereotype is slowly dissipating.”
Jana: I’ll give you a challenge that is me-specific, though I don’t think I’m singular in this. I like to think through things a little bit more than others. It’s not that I won’t contribute in a meeting, but I also like to sit and listen and process. I think that we as women should speak up and say something like, “Hey, guys. We don’t need to make a decision right now. Let’s make sure that we’re thinking through some of these things.” And women’s thought patterns will differ from men’s, but that doesn’t mean that there’s no crossover. So this is more about being an inclusive environment for everyone.
Claudia: I honestly do not see specific challenges that would not apply to other fields or to men as well. In fact, I think that most of the challenges of building a successful career in data science are the same for all.
One component that is often overlooked but very important is communication skills with non-data science folks. This includes the ability to explain data science as well as ask the right questions to deeply understand what the true problem is that needs solving. It might mean that you have to question or even contradict senior management in different business units, which requires political acumen as well as a lot of confidence. I think as women we have a leg up to not appear threatening, but at the same time, we might need to fight a bit harder to be taken seriously. Confidence is key!
Lillian: Women constantly face gender discrimination based on how we look and what we wear. It’s endemic. Employers are even legally allowed to force women to wear makeup, although men cannot be required to do so. We are operating in a system that promotes the underlying message that at least part of our value is in how we look. But then, if a woman is too pretty, people will want her to do extra things to look less attractive. We face a huge control issue, where society (even including other women) is continually judging and controlling us based on our level of attractiveness. It’s pretty sad and irrelevant. This is true for data science as it is for any other field, but in data science, there is more pressure because there are fewer women.
Jennifer: Having enough street cred. I don’t know why this is, but there is a perception that if you are a woman with a manicure and wear jewelry, then you must not know how to program or understand advanced analytics. But a well-groomed man carrying a cappuccino cup gets immediate respect. I don’t get it. I think that stereotype is slowly dissipating.
Sarah: It is tough for me to generalize. I do not want to assume that we are stereotyped, but I have had my awkward moments. That being said, I imagine my male counterparts have also experienced many such moments for a variety of reasons. Personally, I know that I need to avoid the tendency to fixate on why I may have been singled out or read into comments too deeply and assume they are because I am a woman. I think it’s easy to allow yourself to feel that way. My approach is certainly not to try to dress differently or act a certain way. Once I was revealed to an imposter—in other words, not true to myself—I will only feel worse. I think it’s important to celebrate your differences and not become defensive and jump to conclusions. I am happy to say, though, that such occasions are rare.
How important are mentors for female professionals? Did you have any mentors that had an impact on your success?
“Think of your mentors as more of a mentor melting pot, and it’s OK to throw a few radicals in there now and then.”
Jana: I often see people seeking that one perfect mentor. But I feel very lucky that I had an amazing group of mentors. As a CEO, I’ve got lots of hats to wear. So, I need a sales mentor, a marketing mentor, a how-do-I-handle-the-board mentor, a how-do-I-be-a-better-leader mentor. Never in my entire career have I had the one person that I’m like, “I want to be like them.” I respect people’s multiple facets and that they are often better at one thing than the other. Don’t be afraid to look to different people for different things.
Think of your mentors as more of a mentor melting pot, and it’s OK to throw a few radicals in there now and then.
Claudia: Mentors are one of the keys to success for anybody. But mentorship does not have to be a formal program and often does not even feel like mentorship. I have had the luck, and maybe good judgment, to work for people who ultimately turned out to be great mentors. I was told that I do not have a huge appreciation for authority. That is not to say that I do not respect authority but that I perceive my boss primarily as a collaborator and often a friend who has my best interests in mind. I am sure this is not true in all managing relationships, but it was in mine. As a result, I was encouraged to grow into the best I can be.
Jennifer: Mentors are definitely important. To find someone who will look out for your best interests when you are not in the room, someone who will praise you publicly and then pull you behind closed doors and tell you all the things that you did wrong and then take you out for coffee… those people are golden. Indispensable. I did have mentors, all of whom were men. I don’t think that mentors for young women have to be other women—just someone who is willing to provide support and guidance.
Sarah: I think it is critical to find a mentor early on. One of the main challenges is that mentors are most effective when they can see themselves in those they are helping. I actually do not believe that this means women should only be mentored by women. Instead, I think it has to do with the path that you have taken, so mentors can recognize and help with challenges they faced themselves. I have had numerous mentors in my career, both academic and professional. They were not always in my own discipline, and I worked for some and not for others, but they each had such deep and lasting effects on my life and career. I think they can be found in very unexpected places if you allow yourself to listen to what they have to say.
(Note: Answers have been edited for clarity and brevity.)
Undergraduate STEM resources:
- Compare Online Data Science Bachelor’s Degrees
- Compare Online Computer Science Bachelor’s Degrees
- How to Get into Data Science
- Why You Need a Data Science Mentor
- A Guide to Finding and Applying to STEM Scholarships
- Exploring a Career in Numbers
- What is the Difference Between Data Science and Computer Science?
- Sponsored: Online Bachelor’s in Computer Science from Simmons University