Data Science in Energy
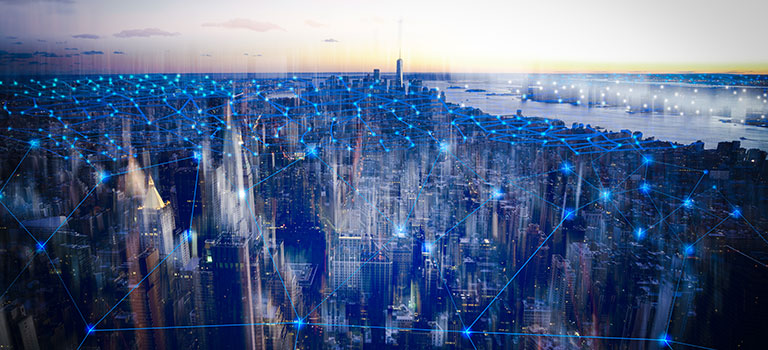
Opportunities in Energy Data Science
The Promise of Big Data
The energy industry has data to work with. Information streams in from different sources – exploration, production, transportation and distribution – and businesses may be struggling to organize it.
Analyzed correctly, big data has the potential to help the industry:
- Discover new energy sources
- Save money on drilling and exploration
- Increase efficiency and productivity
- Predict and stop accidents before they happen
- Avoid power outages
- Gauge consumption patterns
- Match supply to demand
- Plan for better maintenance and repairs
Sponsored Schools
Case Western Reserve University
CWRU Data Analytics Boot Camp
CWRU Data Analytics Boot Camp is a rigorous, part-time program that prepares students with the fundamental skills for data analytics and visualization. Through hands-on, in-person instruction, you’ll cover a wide range of topics and graduate ready to apply your skills in the workforce.
Columbia University
Columbia Engineering Data Analytics Boot Camp
Are you ready to become a data-driven professional? Columbia Engineering Data Analytics Boot Camp is a challenging, part-time bootcamp that equips learners with the specialized skills for data analytics and visualization through hands-on, in-person classes.
University of Texas at Austin
The Data Analysis & Visualization Boot Camp at Texas McCombs
The Data Analysis and Visualization Boot Camp at Texas McCombs puts the student experience first, teaching the knowledge and skills to conduct data analysis on a wide array of real-world problems. Students dive into a comprehensive curriculum, learning how to collect, analyze, and visualize big data.
SPONSORED
Exploration and Discovery
So you’ve got 2D, 3D, 4D seismic monitoring data points. First, you may determine where to look for new oil or gas fields. You may even notice potentially productive areas via seismic trace signatures right in your current fields.
Once you’ve made your discovery, you’ll likely need to assess the likelihood that it will be profitable. Data can help: a well’s historical production along with local drilling, weather and environmental data (e.g., ocean currents for offshore rigs). The result is a much clearer picture of what you have to work with.
Digital Oilfields
Imagine an oilfield where every single piece of equipment was relaying a constant stream of data back to headquarters. Smart rigs would inform operators so they can maintain production flows. Sensors would alert workers about wells in need of repairs. Compressors would warn staff when they’re in danger of overloading.Think it’s a bit sci-fi? Wrong. It’s already been happening for several years.
Think it’s a bit sci-fi? Wrong. It’s already happening. Chevron calls it the “i-field”, BP the “Field of the Future”, and Royal Dutch Shell a “Smart Field.” Many simply call it the digital oilfield.
By combining sensor information (e.g., pressure, temperature, volume, shock and vibration data) with real-time data analytics and high-speed international communications, oil companies can monitor every step of the production process – from initial extraction to daily maintenance.
The aim is to squeeze every last drop of black gold from their investment.
Accident Prevention
The hope is that energy data science can help to stop disasters before they happen.
Sensors help companies monitor the life cycle of each component and catch problems early:
- Are pressure and temperature surging? Enact safety measures.
- Is drilling about to shatter a fragile environmental barrier? Shut it down.
- Will a key piece of equipment need new parts soon? Have them ready and waiting.
It also goes beyond sensors. Data can be harvested from weather forecasts, geologic surveys, maintenance reports, video feeds – you name it – to detect unusual patterns, identify red-light situations and create a clearer picture of risk.
- Are keywords like “leakage” or “vibration” clustering in a single area? Zero in on the problem.
- Have algorithms detected a security breach overseas? Locate the culprit.
In an industry where millions can be affected by a single mistake, these steps aren’t optional.
Clean Energy
Helping the environment can also help the bottom line, and certainly helps job prospects for data scientists. Renewable energy sources like wind, solar and tides are hot and getting hotter.
Take wind. Like oil rigs, wind turbines are made up of many moving parts. Sensors on these parts generate data on everything from wind speed to pitch and yawn degrees. They inform monitors if the turbine is working at peak performance, whether machinery needs maintenance, and if failure is imminent.
Add condition monitoring data (e.g., vibration monitoring, acoustic emissions), work order data (e.g., repair costs) and historical data (25 years and counting) and you have a lot of places to find efficiencies and cut costs.
Even utilities have something to smile about. Although wind and solar are notoriously fluctuating sources of energy, smart grids are helping companies manage the uncertainties. As tidal and solar improve in output and storage, so too will the big data technologies used to monitor and administer to their working parts.
Data Risks
The Challenges Ahead
So what’s stopping the U.S. from having the most efficient, data-driven energy industry in the world? A few things.
1. Disorganization
I’ll step aside and let Jamal Khawaja sum up data inefficiencies:
“Many organizations admit that they are not making the most of the information assets they have residing in structured repositories, and hardly any are exploiting the data held outside of structured systems to any significant degree. So let me frame the problem: it’s not Big Data that is confounding CIOs; it’s deriving value from that data.”
Energy companies know they have huge volumes of data going to waste. They just don’t know how to handle it.
It’s not surprising. The volume, velocity, veracity and variety (the 4Vs) of big data – especially in an industry with so many moving parts – can stagger any data scientist. Finding ways to transform this information into actionable insights is not going to be easy.
2. Variations in Data
Energy has a related problem. Its data sources are all over the map. Here’s Khawaja again:
Data science is rapidly finding ways to overcome these problems, but for the moment, corralling diverse sources remains a challenge.
3. Lack of Data Expertise
This may be the biggest problem. And a most interesting one, too, for career-seekers in the next 20 years. You see, the energy industry is going to need analysts to help uncover answers lurking in their data. The role may confer prestige and status on its holders, both in organizations and in society.
After all, we’re not just talking about boosting a retail store’s quarterly profits. This is about keeping the lifeblood of the future flowing smoothly.
History of Data Analysis and Energy
“Energy forecasting is easy. It’s getting it right that’s difficult.” – Graham Stein, 1996
On August 27, 1859, after a series of setbacks and frustrations, Edwin Drake and his driller, Billy Smith, halted work for the day. Having punched through gravel and over thirty feet of bedrock, their drill bit was now over 69 feet below the surface.
Drake had been hired by Seneca Oil of Connecticut to investigate intriguing deposits near Titusville, Pennsylvania. He, in turn, employed Smith, an expert in drilling for salt.
It could have come to naught. But on the morning of August 28, Smith saw something he’d never seen there before. Something that would spark a boom in drilling, entrepreneurship and data science over the next century: a bubbling sludge of crude oil.
What was so special about that puddle of gurgling goop? Blame it on improving technology and economics.
The Wild Years of Exploration and Discovery
During the late 19th century, oil was in high demand. The country demanded light.
But in those days, striking oil was a hit-and-miss prospect. Methods were haphazard. Many petroleum prospectors sunk their wells in places near known oil and gas seeps and hoped for the best.
As the new century dawned, demand remained insatiable. The oil industry turned its attention from kerosene to gasoline (once considered a useless byproduct) for automobiles and airplanes. There was a lot of money, a lot of guesswork and a lot of waste.
A Seismic Shift in Data Collection
Leading up to and during the 1920s, oil companies began to realize that science could solve some of their problems. Geologists like Wallace Pratt and J. Clarence Karcher were employed to conduct studies on potential oil fields and investigate new tools and techniques.
One of these tools was the seismograph. Originally developed to monitor earthquakes, the seismograph had another handy purpose. By generating small explosions, typically with dynamite, geologists could detect how seismic waves were behaving under the surface of the earth.
From the data collected, scientists could then create a detailed map of the subsurface, including the shape and position of underground rock layers. Combined with data from surface geologic tests, this “seismic reflection profile” would give companies a much better sense of where to drill.
The era of big data in energy had begun.
Farewell Slide Rules, Hello Computers
World War II brought with it new uses for petroleum and natural gas products (e.g., TNT and artificial rubber) as well as the initial development of supercomputers. By this time, oil companies were busily hoarding data from an increasing variety of investigative engineering techniques, including magnetometers and well logging.
In 1963, Humble Oil Company developed new 3D seismic technology. According to Exxon, this data-heavy technology – coupled with the use of massively parallel computers in seismic imaging – helped to sharply reduce finding costs from the 1980s onward.
At the same time, the renewable energy sector was beginning to find its feet. Recognizing future needs, the government and start-up companies began to experiment with wind and solar. The first large commercial electricity-generating wind turbines appeared in 1980s, the federal government installed 1,000s of them in California. And naturally, all that research and development generated even more data.
New Technologies, New Booms
Meanwhile, computers were shrinking, but their power was increasing exponentially. Developments in hardware and software, and improved graphics – not to mention the advent of the Internet – enabled engineers to contemplate boldly going where no drill had gone before.
This was good news for petroleum companies, operating in a worldwide landscape where demand was high but supply appeared to be dwindling.
By the mid-1990s, scientists working as part of a Statoil-Schlumberger joint project were able to use 4D seismic monitoring – analysis of 3D seismic data captured at different times in the same area of an oil field – to differentiate between drained and undrained areas and identify the remaining pockets of oil and gas.
Other searchers applied technical advancements in horizontal drilling and hydraulic fracturing to extract natural gas. In 1997, after a series of failed experiments, Mitchell Energy completed the first economically viable fracture of Texas’s Barnett Shale using slick-water fracturing. This touched off a U.S. rush on natural gas, as well as an avalanche of data, that have yet to subside.
Last updated: June 2020