Data Science in Utilities
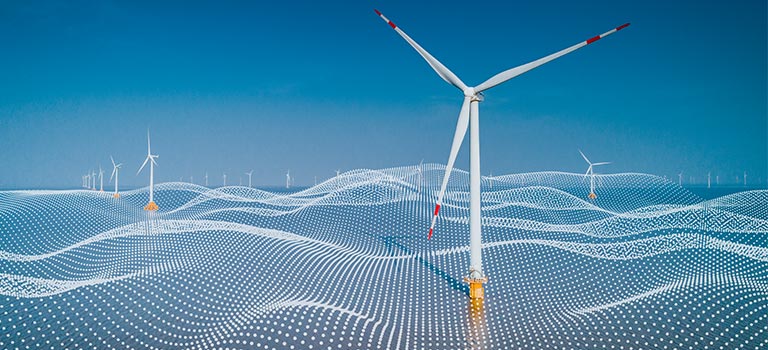
Opportunities in Utility Data Science
The Promise of Big Data
Efforts by the government and utilities to modernize infrastructures are bearing fruit:
- Advanced Metering Infrastructures are generating real-time energy usage data for 40% of United States households
- Utilities have been pouring funds into synchrophasors, or phasor monitoring units (PMUs). PMUs collect measurements such as voltage every second.
Combine this with:
- Historical, in-house customer datasets
- Outage management system reports
- Data from social media, online forms and customer calls
- Video, photo and mapping
- Not to mention publicly available sources (e.g., weather, credit, market)
All this may give you an idea of what data analysts mean by “big” data.
Now, if only utilities can find a way to:
- Harness and process the velocity, variety, volume and veracity of these data
- Invest in the systems, including cloud-based components, as well as the experts needed to understand them
- And commit to changing old operational habits
Sponsored Schools
Case Western Reserve University
CWRU Data Analytics Boot Camp
CWRU Data Analytics Boot Camp is a rigorous, part-time program that prepares students with the fundamental skills for data analytics and visualization. Through hands-on, in-person instruction, you’ll cover a wide range of topics and graduate ready to apply your skills in the workforce.
Columbia University
Columbia Engineering Data Analytics Boot Camp
Are you ready to become a data-driven professional? Columbia Engineering Data Analytics Boot Camp is a challenging, part-time bootcamp that equips learners with the specialized skills for data analytics and visualization through hands-on, in-person classes.
University of Texas at Austin
The Data Analysis & Visualization Boot Camp at Texas McCombs
The Data Analysis and Visualization Boot Camp at Texas McCombs puts the student experience first, teaching the knowledge and skills to conduct data analysis on a wide array of real-world problems. Students dive into a comprehensive curriculum, learning how to collect, analyze, and visualize big data.
SPONSORED
The Impact of Smart Grid Technology
Smart Grids have helped utilities go from being reactive to proactive.
Utilities now have the technology to:
- Improve their monitoring and forecasts of energy consumption
- Manage energy procurement with greater precision
- Pinpoint inefficiencies at macro (e.g., city-wide) and micro (e.g., household) levels
- Predict potential power outages and equipment failures
- Hone their customer segmentation and tailor their offerings based on customer behavior
- Drastically reduce their operational costs
Accurate data predictions can also boost a utility’s performance on settlement markets.
Optimizing Efficiency and Forecasting Consumption
Utility data scientists create ways to maximize operational efficiencies and predict future consumption.
Forecasting is important for utilities that are diversifying their portfolios to include renewable – and fluctuating – energy sources (e.g., wind, solar and tidal). Simulations and models make it possible to determine when energy will be needed and at what level.
Managing Asset Health
Utility data scientists are uniting asset data in a single system and using analysis to identify equipment, networks or pipelines that are headed for failure. This predictive approach also enables them to intelligently budget for replacements.
To get a comprehensive picture of an asset, data scientists may draw on:
- Real-time sensor data
- Historical data
- Operating history
- Maintenance reports
- Technician notes
- Flyover data from drones
- Predictive models (e.g., expected earthquake effects)
- Public datasets (e.g., weather reports)
- And more
Outage Management and Restoration
According to General Electric (GE), outages cost the U.S. economy $150 billion per year. For many businesses and the government, those figures are too high.
Big data to the rescue. With information from smart grids, sensors, historical data, the Internet (e.g., twitter feeds) and asset health systems, utilities can:
- Respond to outages quickly and efficiently
- Avoid them completely
For instance, if they know a storm is on the horizon, utilities may use predictive models to pinpoint potential areas in the line of fire; divert utility crews to hot spots and services to shelters; and warn municipalities and customers.
If an outage does occur, the smart grid will report it immediately. Utility data scientists can then employ big data to:
- Verify, isolate and assess the damage
- Comb internal sources and the Internet for updates on real-time conditions
- Analyze drone and spatial data to identify obstacles (e.g., downed lines, floods, blocked roads)
- Broadcast customer information on social media (e.g., restoration efforts, timeline to power resumption)
Customer Behavior and Engagement
Data scientists can tap into all kinds of sources – smart meters, call centers, social media, billing systems, mobile apps, etc. – to discover more about their customers. Cleaned up and analyzed, these data can help companies:
- Understand consumption trends
- Tailor rates more effectively
- Identify those who are eligible for efficiency or demand-response programs
- Soothe unhappy customers and reinforce loyalty
- Educate individuals and businesses about steps to reduce consumption
In addition to monitoring call centers with sentiment analysis and searching social media and emails for customer opinions, they’re providing:
- Website tools and mobile apps to help customers budget for monthly usage
- Variable-rate structures that put usage into the hands of customers
- Texts or emails when consumption is nearing a target budget
- Consumption graphs and comparison statistics (“you vs. your neighbors”) on monthly bills
- Instant responses to questions on Twitter
- Text or phone messages with outage notifications and updates
Data Risks and Regulations
The Challenges Ahead
The utility industry isn’t setting off celebration fireworks just yet. Creating a data-driven company comes with its own set of challenges.
For utilities, these include:
- Ignorance – Many CEOs are finding it difficult to understand the costs of, and requirements for, implementing big-data solutions. Nobody wants to waste money on systems that may be outdated in a year.
- Apathy – U.S. utilities are largely regulated. Without fierce competition, interest in investing in data science and analytics solutions could weaken.
- Complexity – Utilities and grids are grappling with increasingly complex real-time events. Distributed energy resources and new technologies are further complicating matters.
Data scientists who enter the utilities field may find themselves confronting data locked in siloed systems or the lack of a single, centralized data platform. Integrating existing systems can be difficult in and of itself.
A Matter of Privacy
Then, of course, there’s the not-so-small issue of privacy. With their giant data warehouses full of personal information, utilities are vulnerable to hackers.
A quick Google search will show you that utility data breaches are happening all over the country – from Georgia to Oregon.
It gets worse. The more sources utilities draw upon for their data, the greater the likelihood of customer blowback
- Smart meters have already prompted complaints about safety and unwarranted data usage.
- Utilities using sentiment analysis (e.g., social media) run the risk of being accused of privacy invasion.
History of Data Analysis and Utilities
“Here is our poetry, for we have pulled down the stars to our will.” – Ezra Pound
In June of 1910, a young poet arrived at the docks of New York City. By day, he would harangue the architects of the New York Public Library. By night, he would gaze at the skyscrapers ablaze with electric light
A couple of years later, Ezra Pound put his impressions to paper:
“Squares after squares of flame, set and cut into the ether. Here is our poetry, for we have pulled down the stars to our will.”
It was a relatively new sight – electric lights in skyscraper windows. Poetically beautiful? Yes, but on the mundane level, that electricity still had to be monitored, managed, and paid for. And that requires data. Where did the modern analysis of utilities data begin?
Samuel Insull’s Light Bulb Moment
Chicago in the 1890s. Samuel Insull, president of the Chicago Edison company, was facing a conundrum. To meet peak power demand at dinner time, the company had purchased a lot of power-generating equipment. But this generating capacity was practically all wasted during off-peak periods.
Insull came up with a pricing plan that took into account not only total consumption, but also the timing of that consumption. Businesses like street railways and ice houses were offered low rates for off-peak usage, while customers using power during peak times paid more.
The company’s load factor – the average power divided by the maximum load in each time period – surged. Low rates attracted new customers. Insull’s business was charged to a new level – thanks to a strategic use of data.
The Early Days
As the 20th century progressed, utilities grew to be massive. And customer data grew right along with them.
Local power grids morphed into giant, interconnected systems with huge load centers and high-capacity power lines. To keep up with the ballooning data, in 1965, W.A. Duncan of Kentucky Utilities brought online the electric utility industry’s first all-digital system operation computer – the Westinghouse PRODAC 510.
Nevertheless, due to limited data collection and processing, the industry was still working with estimates:
- Fixed and dual tariffs were the norm; nighttime power was generally charged at a lower rate than daytime.
- Meters, which had been around since the 1880s, continued to be manually checked. Monthly water bills might be estimated from one annual reading.
- Peak prices were averaged out and passed along to consumers equally.
Energy demand continued to increase, however, and during the 1970s, unprecedented usage levels led to a series of blackouts, power cuts and brownouts. Clearly, a little innovation was called for. Power loads and usage data were not about to go away.
Automatic Meter Reading (AMR): First Steps In Automation
Enter Ted Paraskevakos. During the late 1960s and early 1970s, this Greek inventor perfected a method for transferring electronic data (e.g., a phone number) via a telephone line. He dubbed it “Caller ID.”
Paraskevakos’s original idea eventually morphed into a new invention, a sensor monitoring system that used digital transmission to read utility meters. In 1977, Metretek, Inc. – the first commercially available, fully automated meter reading and load management system – made its debut, using IBM’s series 1 mini-computer.
Suddenly, utilities had a constant stream of data from automatic meter readings. This information could be stored and analyzed as a company wished. Utility data analysts were now – pun intended – cooking with gas.
There was more to come, though. Energy usage continued to grow exponentially. To keep up, engineers needed increasingly powerful equipment to deliver and track it. And of course, that meant a flood of data to deal with.
Advanced Metering Infrastructure (AMI): Remote Management
Throughout the 1980s, the utility industry continued to refine its Automated Meter Reading (AMR) technology. In addition to electronic meter readings, engineers now added capabilities like alarms (for tampering, leak detection, low battery, etc.), interval data and log meter events.
By the 1990s, AMR was supplanted by Advanced Metering Infrastructure (AMI). AMI brought smart meters capable of programmed data collection and remote reporting on command. Two-way, continuous communication with a central system had arrived on the scene.
Now utilities could monitor electricity usage data in real time. Demand forecasting, demand response, and flow monitoring made it possible to more intelligently conserve energy and to take rapid emergency action.
In 2000, Enel deployed its Telegestore Project. Using smart meters connected via low bandwidth power lines, this remote management system linked 27 million homes across Italy.
It was also at about this time when the term “smart grid” appeared. Smart grids manage and optimize electricity distribution by automatically analyzing data about customer usage and energy loads. Naturally, even more sophisticated sensing and quality management technology soon followed, producing yet more data.
Sensors and Synchrophasors: Automated Quality
Utility companies at this time helped increase electricity quality and reliability. A great way to do this was by quickly detecting anomalies in quality throughout a power grid by means of synchronized detection and comparison. These synchronized measurements are carried out with devices called synchrophasors.
The 2012 book, Smart Grid Communications and Networking, describes a project led by the Bonneville Power Administration (BPA), a federal agency based in the Pacific Northwest. Beginning in the early 1990s, BPA started installing a network of sensors on its grid to enable high-speed monitoring and control of extensive geographic areas using synchrophasors.
It succeeded. In 2000, BPA became the the first utility to implement a comprehensive adoption of synchrophasors in its Wide Area Measurement System (WAMS). Several other similar projects are underway, as of early 2014, yielding, of course, an ocean of data.
Last updated: June 2020