What Is Data Governance and How Does It Work?
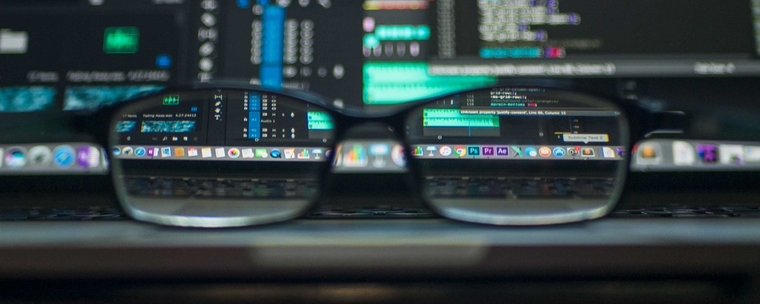
Data governance describes the systems and rules that ensure data is well-organized, accessible, valuable and safe. Data governance is not just one task managed by one type of employee, but rather a wide range of tasks and regulations carried out and monitored by a variety of people.
This makes sense when you consider that much of the reason that data needs to be “governed” is because the store of data is often vast and varied, and the process of managing it and maintaining its full potential is extremely complex. Data governance is an umbrella term for a large-scale operation that involves many moving parts. Major aspects of data governance include:
- Quality-assurance of data
- Consistency of data
- Security of data
- Data management
- Accessibility and usability of data
Data governance is an important part of data science, which ensures that the target data is being cultivated and used to its full potential. However, for a business immersed in data science, it would be a mistake to view data governance as a luxury or “going the extra mile.” For such businesses, data governance is necessary to maintain business operations and keep information secure. Failing to do so can result in consequences ranging from large-scale productivity loss to litigation.
How Data Governance Works
For many businesses—especially in today’s world of emerging machine learning capabilities—it is vital that data is considered an asset. Therefore, protecting data and ensuring its quality should be of great interest to companies that deal in data science, because it is important to their stakeholders and affects profits.
The Benefits of Data Governance
There are many benefits to data governance, all of which have a positive effect on a company’s bottom line, including:
- An improved data pool
- Better use of data
- Consistent data storage practices
- Consistent quality of products and services
- Improved data security
- Improved interdepartmental communication about data
- Easier consumer access to and usage of public-facing data and related services
Because data governance can increase profits, the field offers lucrative careers for people with an educational background in a relevant discipline, such as a data science degree or an information systems degree.
Data Governance vs. Data Management
Data management is an aspect of data governance. Data management refers to the tools, strategies and best practices a company uses to consolidate and organize data. Data governance deals with a much wider scope of data administration that dictates not only how data is managed, but also how to ensure its quality and usability, from the point of acquisition to the end-goal of customer usage.
Without good data management, a company’s valuable data is like a room full of loose documents without any filing system. But without good data governance, it is not only as though there’s no filing system, but as if people are throwing documents in that room that don’t even belong there. No one can walk around. There are sticky notes on some documents with no context. Several different people try to introduce order, but don’t communicate with each other. Occasionally someone walks in off the street and shoves some of the documents in a duffle bag. And who knows what will happen if you try to take a handful of this mess and make something useful out of it.
Data Governance Models
Data governance is a daunting task, but often very necessary. Luckily, you don’t have to approach it blindly. There are many tried-and-true data governance models you can use as a foundation for your own unique data governance strategies.
Traditional Model
In a traditional model of data governance, those who will operate in a governance role will be either identified or assigned their role based on existing managerial roles. The identified governance roles are not usually explicitly stated or defined.
Command-and-Control Model
In the command-and-control model, governance roles are explicitly issued to individuals independent of their existing duties. These people are specifically responsible for duties that fall under the purview of their role.
Non-Invasive Model
In the non-invasive model, governance roles are identified or assigned based on how closely the data and its management relates to the employee’s pre-existing duties. Their new role is explicitly stated and cultivated.
Decentralized vs. Centralized Governance
Decentralized data governance is a model that involves individuals or groups managing their own portion of the overall data pool. Although these individuals or groups likely communicate with each other, they are specifically responsible for their individual portion of the data pool. Centralized governance is a model in which data is compiled and assigned to a single group or company, which processes and manages all the data. Decentralized data governance is usually used in smaller businesses, while centralized data governance is typically employed by larger business operations.
Steps to Creating a Data Governance Framework
To build a successful data governance system for your business, it will be helpful to first build a framework unique to the goals and needs of your company. The following steps will help outline a data governance framework:
- Prioritize areas of improvement.
- Establish data gathering processes and structures.
- Create formal roles and responsibilities for all stakeholders.
- Develop a feedback process for further improvement.
- Ensure the integrity of your data.
Best Practices to Follow
It is important to keep your primary goals and KPIs in mind when trying to develop an effective data governance strategy. The selected goals should explicitly reflect and prioritize ethical data usage. With countless options for data governance, allowing these variables to inform your strategy and structure will give your business guidance on making decisions about your data governance framework.